Tyler Vigen has a great website Spurious Correlations that shows graphs of exactly that.
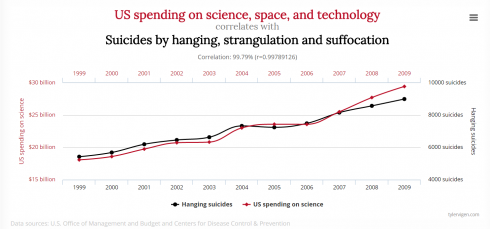
Great for explaining what correlation means, and why correlation does not necessarily mean causation.
Middle and High School … from a Montessori Point of View
Tyler Vigen has a great website Spurious Correlations that shows graphs of exactly that.
Great for explaining what correlation means, and why correlation does not necessarily mean causation.
A nice animation showing the inner workings of a cell. There is a narrated version.
Previously, I showed how to solve a simple problem of motion at a constant velocity analytically and numerically. Because of the nature of the problem both solutions gave the same result. Now we’ll try a constant acceleration problem which should highlight some of the key differences between the two approaches, particularly the tradeoffs you must make when using numerical approaches.
The Problem
Analytical Solution
We know that acceleration (a) is the change in velocity with time (t):
so if we integrate acceleration we can find the velocity. Then, as we saw before, velocity (v) is the change in position with time:
which can be integrated to find the position (x) as a function of time.
So, to summarize, to find position as a function of time given only an acceleration, we need to integrate twice: first to get velocity then to get x.
For this problem where the acceleration is a constant 0.2 m/s2 we start with acceleration:
which integrates to give the general solution,
To find the constant of integration we refer to the original question which does not say anything about velocity, so we assume that the initial velocity was 0: i.e.:
at t = 0 we have v = 0;
which we can substitute into the velocity equation to find that, for this problem, c is zero:
making the specific velocity equation:
we replace v with dx/dt and integrate:
This constant of integration can be found since we know that the ball starts at the origin so
at t = 0 we have x = 0, so;
Therefore our final equation for x is:
To summarize the analytical solution:
These are all a function of time so it might be more proper to write them as:
Velocity and acceleration represent rates of change which so we could also write these equations as:
or we could even write acceleration as the second differential of the position:
or, if we preferred, we could even write it in prime notation for the differentials:
As we saw before we can determine the position of a moving object if we know its old position (xold) and how much that position has changed (dx).
where the change in position is determined from the fact that velocity (v) is the change in position with time (dx/dt):
which rearranges to:
So to find the new position of an object across a timestep we need two equations:
In this problem we don’t yet have the velocity because it changes with time, but we could use the exact same logic to find velocity since acceleration (a) is the change in velocity with time (dv/dt):
which rearranges to:
and knowing the change in velocity (dv) we can find the velocity using:
Therefore, we have four equations to find the position of an accelerating object (note that in the third equation I’ve replaced v with vnew which is calculated in the second equation):
These we can plug into a python program just so:
motion-01-both.py
from visual import * # Initialize x = 0.0 v = 0.0 a = 0.2 dt = 1.0 # Time loop for t in arange(dt, 20+dt, dt): # Analytical solution x_a = 0.1 * t**2 # Numerical solution dv = a * dt v = v + dv dx = v * dt x = x + dx # Output print t, x_a, x
which give output of:
>>> 1.0 0.1 0.2 2.0 0.4 0.6 3.0 0.9 1.2 4.0 1.6 2.0 5.0 2.5 3.0 6.0 3.6 4.2 7.0 4.9 5.6 8.0 6.4 7.2 9.0 8.1 9.0 10.0 10.0 11.0 11.0 12.1 13.2 12.0 14.4 15.6 13.0 16.9 18.2 14.0 19.6 21.0 15.0 22.5 24.0 16.0 25.6 27.2 17.0 28.9 30.6 18.0 32.4 34.2 19.0 36.1 38.0 20.0 40.0 42.0
Here, unlike the case with constant velocity, the two methods give slightly different results. The analytical solution is the correct one, so we’ll use it for reference. The numerical solution is off because it does not fully account for the continuous nature of the acceleration: we update the velocity ever timestep (every 1 second), so the velocity changes in chunks.
To get a better result we can reduce the timestep. Using dt = 0.1 gives final results of:
18.8 35.344 35.532 18.9 35.721 35.91 19.0 36.1 36.29 19.1 36.481 36.672 19.2 36.864 37.056 19.3 37.249 37.442 19.4 37.636 37.83 19.5 38.025 38.22 19.6 38.416 38.612 19.7 38.809 39.006 19.8 39.204 39.402 19.9 39.601 39.8 20.0 40.0 40.2
which is much closer, but requires a bit more runtime on the computer. And this is the key tradeoff with numerical solutions: greater accuracy requires smaller timesteps which results in longer runtimes on the computer.
To generate a graph of the data use the code:
from visual import * from visual.graph import * # Initialize x = 0.0 v = 0.0 a = 0.2 dt = 1.0 analyticCurve = gcurve(color=color.red) numericCurve = gcurve(color=color.yellow) # Time loop for t in arange(dt, 20+dt, dt): # Analytical solution x_a = 0.1 * t**2 # Numerical solution dv = a * dt v = v + dv dx = v * dt x = x + dx # Output print t, x_a, x analyticCurve.plot(pos=(t, x_a)) numericCurve.plot(pos=(t,x))
which gives:
We’ve started working on the physics of motion in my programming class, and really it boils down to solving differential equations using numerical methods. Since the class has a calculus co-requisite I thought a good way to approach teaching this would be to first have the solve the basic equations for motion (velocity and acceleration) analytically–using calculus–before we took the numerical approach.
Analytical Solution:
Well, we know that speed is the change in position (in the x direction in this case) with time, so a constant velocity of 0.5 m/s can be written as the differential equation:
To get the ball’s position at a given time we need to integrate this differential equation. It turns out that my calculus students had not gotten to integration yet. So I gave them the 5 minute version, which they were able to pick up pretty quickly since integration’s just the reverse of differentiation, and we were able to move on.
Integrating gives:
which includes a constant of integration (c). This is the general solution to the differential equation. It’s called the general solution because we still can’t use it since we don’t know what c is. We need to find the specific solution for this particular problem.
In order to find c we need to know the actual position of the ball is at one point in time. Fortunately, the problem states that the ball starts at the origin where x=0 so we know that:
So we plug these values into the general solution to get:
solving for c gives:
Therefore our specific solution is simply:
And we can write a simple python program to print out the position of the ball every second for 20 seconds:
motion-01-analytic.py
for t in range(21): x = 0.5 * t print t, x
which gives the result:
>>> 0 0.0 1 0.5 2 1.0 3 1.5 4 2.0 5 2.5 6 3.0 7 3.5 8 4.0 9 4.5 10 5.0 11 5.5 12 6.0 13 6.5 14 7.0 15 7.5 16 8.0 17 8.5 18 9.0 19 9.5 20 10.0
Numerical Solution:
Finding the numerical solution to the differential equation involves not integrating, which is particularly good if the differential equation can’t be integrated.
We start with the same differential equation for velocity:
but instead of trying to solve it we’ll just approximate a solution by recognizing that we use dx/dy to represent when the change in x and t are really, really small. If we were to assume they weren’t infinitesimally small we would rewrite the equations using deltas instead of d’s:
now we can manipulate this equation using algebra to show that:
so the change in the position at any given moment is just the velocity (0.5 m/s) times the timestep. Therefore, to keep track of the position of the ball we need to just add the change in position to the old position of the ball:
Now we can write a program to calculate the position of the ball using this numerical approximation.
motion-01-numeric.py
from visual import * # Initialize x = 0.0 dt = 1.0 # Time loop for t in arange(dt, 21, dt): v = 0.5 dx = v * dt x = x + dx print t, x
I’m sure you’ve noticed a couple inefficiencies in this program. Primarily, that the velocity v, which is a constant, is set inside the loop, which just means it’s reset to the same value every time the loop loops. However, I’m putting it in there because when we get working on acceleration the velocity will change with time.
I also import the visual library (vpython.org) because it imports the numpy library and we’ll be creating and moving 3d balls in a little bit as well.
Finally, the two statements for calculating dx and x could easily be combined into one. I’m only keeping them separate to be consistent with the math described above.
A Program with both Analytical and Numerical Solutions
For constant velocity problems the numerical approach gives the same results as the analytical solution, but that’s most definitely not going to be the case in the future, so to compare the two results more easily we can combine the two programs into one:
motion-01.py
from visual import * # Initialize x = 0.0 dt = 1.0 # Time loop for t in arange(dt, 21, dt): v = 0.5 # Analytical solution x_a = v * t # Numerical solution dx = v * dt x = x + dx # Output print t, x_a, x
which outputs:
>>> 1.0 0.5 0.5 2.0 1.0 1.0 3.0 1.5 1.5 4.0 2.0 2.0 5.0 2.5 2.5 6.0 3.0 3.0 7.0 3.5 3.5 8.0 4.0 4.0 9.0 4.5 4.5 10.0 5.0 5.0 11.0 5.5 5.5 12.0 6.0 6.0 13.0 6.5 6.5 14.0 7.0 7.0 15.0 7.5 7.5 16.0 8.0 8.0 17.0 8.5 8.5 18.0 9.0 9.0 19.0 9.5 9.5 20.0 10.0 10.0
Solving a problem involving acceleration comes next.
After batting around a number of ideas, three of my middle schoolers settled on building a model skate park out of popsicle sticks and cardboard for their interim project.
A lot of hot glue was involved.
The ramps turned out to be pretty easy, but on the second day they decided that the wanted a bowl, which proved to be much more challenging. They cut out sixteen profiles out of thicker cardboard, made a skeleton out of popsicle sticks, and then coated the top with thin, cereal-box cardboard.
When they were done they painted the whole thing grey–to simulate concrete I think–except for the sides, which were a nice flat blue so that they could put their own miniature graffiti over the top.
It was a lot of careful, well thought-out work.
A couple middle-schoolers decided to build a gaga ball pit for their interim project. Since they’d already had their plan improved and I’d picked up the wood over the weekend, they figured they could get it done in a day or two and then move on to other things for the rest of the week. However, it turned out to be a bit more involved.
They spent most of their first day–they just had afternoons to work on this project–figuring out where to build the thing. It’s pretty large, and our head-of-school was in meetings all afternoon, so there was a lot of running back and forth.
The second day involved some math. Figuring out where to put the posts required a little geometry to determine the internal angles of an octagon, and some algebra (including Pythagoras’ Theorem) to calculate the distance across the pit. The sum a2 + a2 proved to be a problem, but now that they’ve had to do it in practice they won’t easily forget that it’s not a4.
Mounting the rails on the posts turned out the be the main challenge on day three. They initially opted for trying to drill the rails in at an angle, but found out pretty quickly that that was going to be extremely difficult. Eventually, they decided to rip the posts at a 45 degree angle to get the 135 degree outer angle they needed. We ran out of battery power for the saw and our lag screws were too long for the new design, so assembly would have to wait another day.
Finally, on day four they put the pit together. It only took about an hour–they’d had the great idea on day three to put screws into the posts at the right height, so that they could rest the rails on the screws to hold them into place temporarily as they mounted the rails. By the time they added the final side the octagon was only off by a few, easily adjusted centimeters.
They did an excellent job and noted, in our debrief, just how important the planning was, even though it was their least favorite part of the project. I’d call it a successful project.
On this year’s trip to the Current River with the Middle School we were able to see outcrops of the three major types of rocks: igneous, metamorphic, and sedimentary.
We stopped by Elephant Rocks State Park on the way down to the river to check out the gorgeous pink granite that makes up the large boulders. The coarse grains of quartz (translucent) interbedded with the pink orthoclase crystals make for an excellent example of a slow-cooling igneous rock.
On the second day out on the canoes we clambered up the rocks in the Prairie Creek valley to see jump into the small waterfall pool. The rocks turned out to look a lot like the granite of Elephant Rocks if the large crystals had been heated up and deformed plastically. This initial stage of the transformation allowed me to talk about metamorphic rocks althought we’ll see some much more typical samples when we get back to the classroom.
We visited a limestone cave on the third day, although we’ve been canoeing through a lot of limestone for on the previous two days. This allowed us to talk about sedimentary rocks: their formation in the ocean and then uplift via tectonic collisions.
Back at camp, we summarized what we saw with a discussion of the rock cycle, using a convergent plate margin as an example. Note: sleeping mats turned out to be excellent models for converging tectonic plates.
Note to self: It might make sense to add extra time at the beginning and end of the trip to do some more geology stops. Johnson Shut-Inns State Park is between Elephant Rocks and Eminence, and we saw a lot of interesting sedimentary outcrops on the way back to school as we headed up to Rolla.
I’m teaching a numerical methods class that’s partly an introduction to programming, and partly a survey of numerical solutions to different types of problems students might encounter in the wild. I thought I’d look into doing a session on genetic algorithms, which are an important precursor to things like networks that have been found to be useful in a wide variety of fields including image and character recognition, stock market prediction and medical diagnostics.
The ai-junkie, bare-essentials page on genetic algorithms seemed a reasonable place to start. The site is definitely readable and I was able to put together a code to try to solve its example problem: to figure out what series of four mathematical operations using only single digits (e.g. +5*3/2-7) would give target number (42 in this example).
The procedure is as follows:
And this is the code I came up with:
genetic_algorithm2.py
''' Write a program to combine the sequence of numbers 0123456789 and the operators */+- to get the target value (42 (as an integer)) ''' ''' Procedure: 1. Randomly generate a few sequences (ns=10) where each sequence is 8 charaters long (ng=8). 2. Check how close the sequence's value is to the target value. The closer the sequence the higher the weight it will get so use: w = 1/(value - target) 3. Chose two of the sequences in a way that gives preference to higher weights. 4. Randomly combine the successful sequences to create new sequences (ns=10) 5. Repeat until target is achieved. ''' from visual import * from visual.graph import * from random import * import operator # MODEL PARAMETERS ns = 100 target_val = 42 #the value the program is trying to achieve sequence_length = 4 # the number of operators in the sequence crossover_rate = 0.3 mutation_rate = 0.1 max_itterations = 400 class operation: def __init__(self, operator = None, number = None, nmin = 0, nmax = 9, type="int"): if operator == None: n = randrange(1,5) if n == 1: self.operator = "+" elif n == 2: self.operator = "-" elif n == 3: self.operator = "/" else: self.operator = "*" else: self.operator = operator if number == None: #generate random number from 0-9 self.number = 0 if self.operator == "/": while self.number == 0: self.number = randrange(nmin, nmax) else: self.number = randrange(nmin, nmax) else: self.number = number self.number = float(self.number) def calc(self, val=0): # perform operation given the input value if self.operator == "+": val += self.number elif self.operator == "-": val -= self.number elif self.operator == "*": val *= self.number elif self.operator == "/": val /= self.number return val class gene: def __init__(self, n_operations = 5, seq = None): #seq is a sequence of operations (see class above) #initalize self.n_operations = n_operations #generate sequence if seq == None: #print "Generating sequence" self.seq = [] self.seq.append(operation(operator="+")) # the default operation is + some number for i in range(n_operations-1): #generate random number self.seq.append(operation()) else: self.seq = seq self.calc_seq() #print "Sequence: ", self.seq def stringify(self): seq = "" for i in self.seq: seq = seq + i.operator + str(i.number) return seq def calc_seq(self): self.val = 0 for i in self.seq: #print i.calc(self.val) self.val = i.calc(self.val) return self.val def crossover(self, ingene, rate): # combine this gene with the ingene at the given rate (between 0 and 1) # of mixing to create two new genes #print "In 1: ", self.stringify() #print "In 2: ", ingene.stringify() new_seq_a = [] new_seq_b = [] for i in range(len(self.seq)): if (random() < rate): # swap new_seq_a.append(ingene.seq[i]) new_seq_b.append(self.seq[i]) else: new_seq_b.append(ingene.seq[i]) new_seq_a.append(self.seq[i]) new_gene_a = gene(seq = new_seq_a) new_gene_b = gene(seq = new_seq_b) #print "Out 1:", new_gene_a.stringify() #print "Out 2:", new_gene_b.stringify() return (new_gene_a, new_gene_b) def mutate(self, mutation_rate): for i in range(1, len(self.seq)): if random() < mutation_rate: self.seq[i] = operation() def weight(target, val): if val <> None: #print abs(target - val) if abs(target - val) <> 0: w = (1. / abs(target - val)) else: w = "Bingo" print "Bingo: target, val = ", target, val else: w = 0. return w def pick_value(weights): #given a series of weights randomly pick one of the sequence accounting for # the values of the weights # sum all the weights (for normalization) total = 0 for i in weights: total += i # make an array of the normalized cumulative totals of the weights. cum_wts = [] ctot = 0.0 cum_wts.append(ctot) for i in range(len(weights)): ctot += weights[i]/total cum_wts.append(ctot) #print cum_wts # get random number and find where it occurs in array n = random() index = randrange(0, len(weights)-1) for i in range(len(cum_wts)-1): #print i, cum_wts[i], n, cum_wts[i+1] if n >= cum_wts[i] and n < cum_wts[i+1]: index = i #print "Picked", i break return index def pick_best(weights): # pick the top two values from the sequences i1 = -1 i2 = -1 max1 = 0. max2 = 0. for i in range(len(weights)): if weights[i] > max1: max2 = max1 max1 = weights[i] i2 = i1 i1 = i elif weights[i] > max2: max2 = weights[i] i2 = i return (i1, i2) # Main loop l_loop = True loop_num = 0 best_gene = None ##test = gene() ##test.print_seq() ##print test.calc_seq() # initialize genes = [] for i in range(ns): genes.append(gene(n_operations=sequence_length)) #print genes[-1].stringify(), genes[-1].val f1 = gcurve(color=color.cyan) while (l_loop and loop_num < max_itterations): loop_num += 1 if (loop_num%10 == 0): print "Loop: ", loop_num # Calculate weights weights = [] for i in range(ns): weights.append(weight(target_val, genes[i].val)) # check for hit on target if weights[-1] == "Bingo": print "Bingo", genes[i].stringify(), genes[i].val l_loop = False best_gene = genes[i] break #print weights if l_loop: # indicate which was the best fit option (highest weight) max_w = 0.0 max_i = -1 for i in range(len(weights)): #print max_w, weights[i] if weights[i] > max_w: max_w = weights[i] max_i = i best_gene = genes[max_i] ## print "Best operation:", max_i, genes[max_i].stringify(), \ ## genes[max_i].val, max_w f1.plot(pos=(loop_num, best_gene.val)) # Pick parent gene sequences for next generation # pick first of the genes using weigths for preference ## index = pick_value(weights) ## print "Picked operation: ", index, genes[index].stringify(), \ ## genes[index].val, weights[index] ## ## # pick second gene ## index2 = index ## while index2 == index: ## index2 = pick_value(weights) ## print "Picked operation 2:", index2, genes[index2].stringify(), \ ## genes[index2].val, weights[index2] ## (index, index2) = pick_best(weights) # Crossover: combine genes to get the new population new_genes = [] for i in range(ns/2): (a,b) = genes[index].crossover(genes[index2], crossover_rate) new_genes.append(a) new_genes.append(b) # Mutate for i in new_genes: i.mutate(mutation_rate) # update genes array genes = [] for i in new_genes: genes.append(i) print print "Best Gene:", best_gene.stringify(), best_gene.val print "Number of iterations:", loop_num ##
When run, the code usually gets a valid answer, but does not always converge: The figure at the top of this post shows it finding a solution after 142 iterations (the solution it found was: +8.0 +8.0 *3.0 -6.0). The code is rough, but is all I have time for at the moment. However, it should be a reasonable starting point if I should decide to discuss these in class.